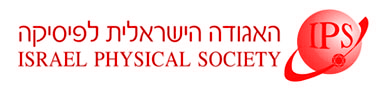
Home
About/Contact
Newsletters
Events/Seminars
2020 IPS Conference
Study Materials
Corporate Members
Home
About/Contact
Newsletters
Events/Seminars
2020 IPS Conference
Study Materials
Corporate Members
Reaction networks frequently appear in many natural systems, such as chemistry, biology and ecology. These networks can be characterized by the time dependant average populations of the different reactive species. The commonly used method to obtain this characterization is based on rate equations models, incorporating the law of mass action kinetics. For macroscopic systems, with typically large populations, this approach is suitable. However, when the system is microscopic, it becomes governed by fluctuations, the law of mass action kinetics no longer applies, and the rate equations fail. To obtain an accurate description of microscopic reaction networks, one must refer to stochastic methods based on the master equation. This becomes a difficult problem because the number of microscopic states in such systems increases exponentially with the number of reactive species. Direct integration of the master equation is thus infeasible and is replaced by Monte Carlo simulations using the Gillespie algorithm. While Monte Carlo simulations are a highly effective tool, equation-based formulations are more amenable to analytical treatment and may provide deeper insight into the dynamics of the network. In this talk we introduce several highly efficient equation-based methods for the analysis of stochastic reaction networks. The methods are based on dimensional reduction of the master equation, in a way that reflects the topological structure of the network, and the stoichiometric nature of the reactions. The number of equations is dramatically reduced, enabling the simulation of highly complex networks, previously considered too elaborate for stochastic modeling. For simple systems, these methods have provided crucial analytical time dependent solutions, whereas for complex systems they were shown to provide highly efficient numerical simulations. Application fields range from astrochemistry, to systems biology and ecology.