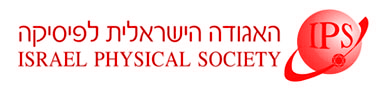
Home
About/Contact
Newsletters
Events/Seminars
2020 IPS Conference
Study Materials
Corporate Members
Home
About/Contact
Newsletters
Events/Seminars
2020 IPS Conference
Study Materials
Corporate Members
In unsupervised training of deep neural networks one often seeks to
reproduce the probability distribution of the dataset. In this talk
I'll discuss a different goal which is to make the log-probability of
the coarse grained dataset as local as possible. I'll argue that this
goal mappable to a certain information-bottleneck problem and also to
the renormalization-group (RG) technique common in physics.
Furthermore I'll present recent analytical results supporting this
explicit link between the information-bottleneck approach and RG and give an outlook on some potential applications in machine learning and
physics.