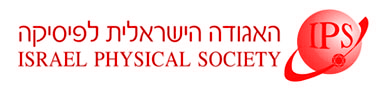
Home
About/Contact
Newsletters
Events/Seminars
2020 IPS Conference
Study Materials
Corporate Members
Home
About/Contact
Newsletters
Events/Seminars
2020 IPS Conference
Study Materials
Corporate Members
Particle flow is an event reconstruction technique, which tries to reconstruct the four-momenta of all the stable particles in an event, using the information from individual sub-detector components. We attempt to use CNN to process layered calorimeter images around the charged inner detector track, in events where a pi0 hit the calorimeter (or photons from a p0 decay) close to the charged track, to identify the neutral energy component. Once established, this method has the potential of much better estimation of neutral energy components, pileup estimation, and jet energy resolution. An autoencoder is used to identify faults in ATLAS sTGC detectors. In particular, cabling errors are very difficult to detect and should be identified before the installation of the detector wheels. We demonstrate with a semi-realistic toy model, how a simple autoencoder NN can easily detect malfunctions in the sTGC boards, from cabling errors to dead detector areas.