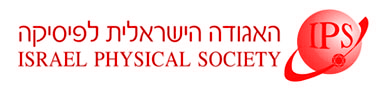
Home
About/Contact
Newsletters
Events/Seminars
2020 IPS Conference
Study Materials
Corporate Members
Home
About/Contact
Newsletters
Events/Seminars
2020 IPS Conference
Study Materials
Corporate Members
Peritoneal dialysis (PD) is a recognized form of treatment for patients with severe chronic kidney failure. Acute peritonitis is a major complication associated with this procedure, while frequent occurrence of peritonitis can be associated with high risk of mortality and morbidity. Diagnosis of peritonitis is heavily dependent on laboratory tests including etiological agents’ detection, but these time-consuming tests are subject to accuracy and sensitivity limitations. In our research, a fast diagnosis of bacterial peritonitis is based on polymorphonuclear leukocytes (PMNs) functional changes that can be assessed by the chemiluminescent (CL) reaction within several hours. For the assessment and discrimination of functional states of PMNs, we have applied a classification algorithm (C4.5) to the time-series data produced by dynamic component chemiluminescence analysis in a luminol-amplified whole blood samples taken from PD patients. Feature extraction is based on the evaluation of CL kinetic patterns of stimulated PMNs. This novel method of combining whole-blood CL, kinetics component analysis, and classification of clinical groups using a decision-tree algorithm has shown a high predictive diagnostic value and it may assist clinicians in detection of PD associated clinical states.