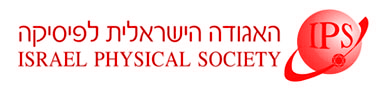
Home
About/Contact
Newsletters
Events/Seminars
2020 IPS Conference
Study Materials
Corporate Members
Home
About/Contact
Newsletters
Events/Seminars
2020 IPS Conference
Study Materials
Corporate Members
Noise and information are deeply related in physical systems. It is of similar interest to understand how the intrinsic noisiness of neural processes affects information storage in the brain. I will focus on a common theoretical model of persistent memory in neural networks, that maintain information about a continuous variable such as an angle or spatial position. We recently showed that a fundamental relationship exists in these networks between two statistical measures of stochasticity: a static measure, which quantifies how well an external observer can infer the value of the stored memory by observing network spikes in a short time interval; and a measure which quantifies the dissipation of memory due to random changes in the network's state. The relationship takes the form of a rigorous inequality, which bounds from below the dissipation of memory. I will discuss several consequences of this inequality, as well as its possible relevance to specific memory networks.