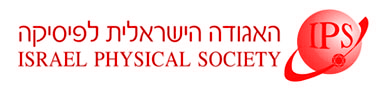
Home
About/Contact
Newsletters
Events/Seminars
2020 IPS Conference
Study Materials
Corporate Members
Home
About/Contact
Newsletters
Events/Seminars
2020 IPS Conference
Study Materials
Corporate Members
We investigate a population of binary mistake sequences that result from learning with parametric models of different order. We obtain estimates of their error, algorithmic complexity and divergence from a purely random Bernoulli sequence. We study the relationship of these variables to the learner's information density parameter which is defined as the ratio between the lengths of the compressed to uncompressed files that contain the learner's decision rule. The results indicate that good learners have a low information density$\rho$ while bad learners have a high $\rho$. Bad learners generate atypically chaotic mistake sequences while good learners generate typically chaotic sequences that divide into two subgroups: the first consists of the typically stochastic sequences (with low divergence) which includes the sequences generated by the Bayes optimal predictor. The second subgroup consists of the atypically stochastic (but still typically chaotic) sequences that deviate from truly random Bernoulli sequences. The learner acts as a static structure which ``scatters'' the bits of an input sequence (to be predicted) proportional to its information density $\rho$ thereby deforming its randomness characteristics.