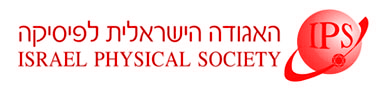
Home
About/Contact
Newsletters
Events/Seminars
2020 IPS Conference
Study Materials
Corporate Members
Home
About/Contact
Newsletters
Events/Seminars
2020 IPS Conference
Study Materials
Corporate Members
In the past decade deep neural networks (DNNs) came to the fore as the leading machine learning algorithms for a variety of tasks. Their raise was founded on market needs and engineering craftsmanship, the latter based more on trial and error than on theory. While still far behind the application forefront, the theoretical study of DNNs has recently made important advancements in analyzing the highly over-parametrized regime where some exact explicit results have been obtained for shallow DNNs. Leveraging these ideas and adopting a more physics-like approach, here we construct a versatile field-theory formalism for supervised deep learning involving renormalization group, Feynmann diagrams, and replicas. We show that our approach leads to accurate analytical predictions for the learning curves of truly deep DNNs trained on toy polynomial regression problems. We further show that it can accurately account for finite-width corrections. Being a condensed system of artificial neurons, we believe that such tools and methodologies borrowed from condensed matter physics would prove essential for obtaining an accurate quantitative understanding of deep learning.